Digital Health vs the Causes of Incidence
We need comparative research at scale to move things forward
We’ve emerged from two weeks of sub-zero weather here in Madison with a frozen lake and unreasonable enthusiasm for twenty degrees. While snowed in, I’ve been enjoying the recent crop of solo drum records, like the latest from Nate Smith, and wishing I could get to Mark Guiliana’s solo shows. Alas.
Over the break, I ran across slides from a presentation I gave at Stanford Medicine X way back in September 2012, making the case that digital health could open a new front on prevention. I’ve been reflecting on how this never really took hold (with a few exceptions) and thought the topic and the work of Geoffrey Rose worth resurrecting. So I converted the deck into this post and added some asthma epidemiology to illustrate the opportunity.
The investigative potential of digital health
Propeller (or Asthmapolis as it was called then) was put together to track asthma. We aimed to create technology that helped people and their families accomplish the work of illness. But we did so primarily because we thought collecting information that made visible its day-to-day burden would revealing something about the disease that could be used to prevent it in the first place. Our work with the city of Louisville was our best example of that effort.
This ambition remains an under-appreciated potential of digital health. In the accumulation of data from new sources and at previously impractical scales, it becomes possible to sneak up on prevalent chronic diseases, catch them in moments of weakness and odd behavior, and deliver insight that can help reduce their prevalence.
It requires technology companies embrace a broader, comparative perspective on health. That means thinking beyond an individual and their physician and the current configuration of healthcare in the US. But it was well described nearly four decades ago, when in 1985, physician-epidemiologist Geoffrey Rose published a paper entitled Sick Individuals and Sick Populations.
Rose's essay became a landmark for clearly demonstrating how the causes of disease in an individual could differ from those that determine the overall rate of disease in a population. He made the point with this famous figure illustrating the distribution of systolic blood pressure in populations of Kenyan nomads and London civil servants.
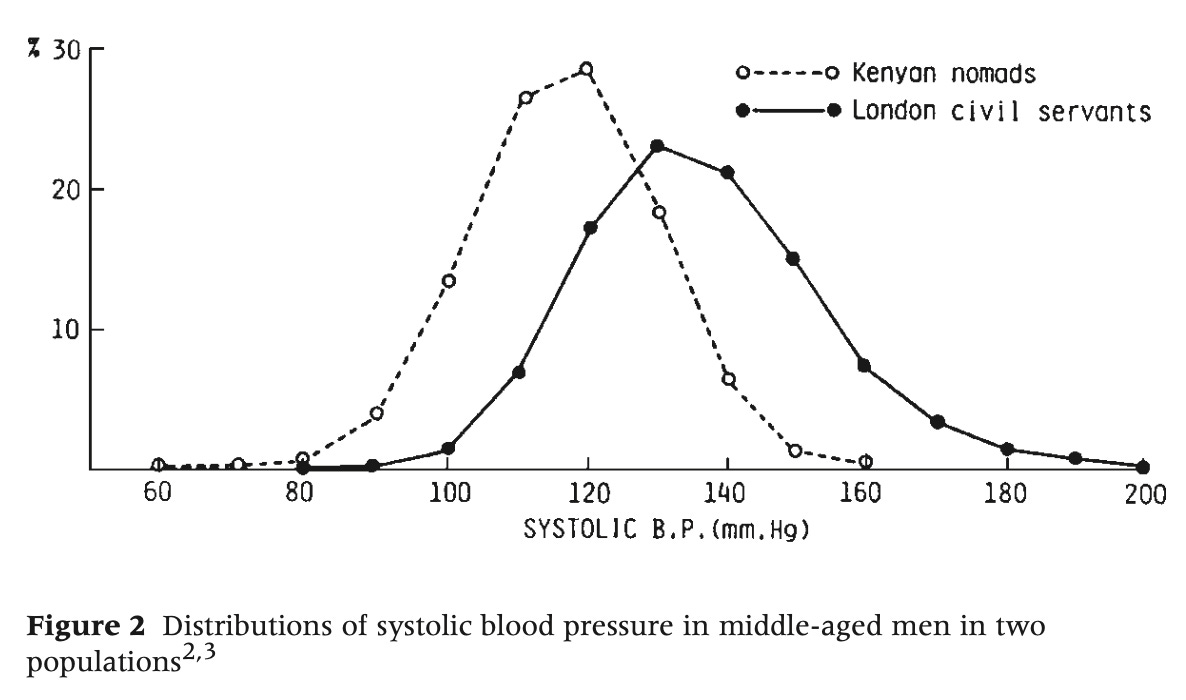
“Why is hypertension absent in Kenya and common in London?”
Rose explained that you could investigate in each population why some individuals had higher blood pressure than others and arrive at the same conclusion. You would probably settle on genetic variation, and find some contribution of environment and behavior. In other words, you could achieve a complete understanding of why individuals within each population varied, and yet miss the most important public health question: Why is hypertension nearly absent in Kenyans and common in Londoners?
The answer to that question, Rose argued, had nothing to do with the characteristics of individuals. Rather, it had to do with some kind of force acting on the population as a whole, shifting the distribution one direction or the other.
His paper provoked epidemiologists to contemplate their dual-sided mission. Did they want to explain why one individual became ill (or stayed healthy), or might they use their techniques to understand and prevent disease in populations?
Digital health has unwittingly labored with the same dilemma. Should the field be content to improve the day-to-day management and quality of life of individuals with a disease, or could we achieve something more significant and meaningful, potentially reducing the prevalence and incidence of diseases in populations?
There are puzzling data and questions in asthma epidemiology that illustrate the differences in these orientations and highlight how digital health can get us closer to those more ambitious goals.
Established risk factors for asthma don't explain prevalence patterns and time trends
For the past four or five decades, the prevalence of asthma has been rising inexorably, almost uniformly, and in nearly every population in the world where it’s been measured. But recently, changing and contrary trends in its epidemiology have challenged a lot of our understanding of the origins of the disease.
Just when we thought we had the evidence and theoretical framework to account for the long rise in asthma cases, now we have to explain why in some populations we appear to be witnessing a decline, while in similar and neighboring ones, wholly different patterns exist.
It’s not easy to untangle. For example, Statistics Canada recently reported that prevalence of asthma in Canadian children had fallen to its lowest level in ten years. But during the same period the United States saw a stabilization of prevalence and then a return to a significant increase.
Official reports from Statistics Canada pointed to improvements in air quality and reductions in tobacco smoke exposure. But the situation is quite a bit more complex than that. Unfortunately, Stats Canada has it wrong, but at least they’re wrong in a way that productively highlights the distinction between the causes of disease in individuals and the causes in populations.
The key difference hinges on the existence of two types of risk factors. Stats Canada, like much of public health, is naturally attracted to secondary risk factors, or things that determine who in a population will develop a disease. Rose called these the “causes of variants.” By contrast, primary risk factors — what Rose termed the “causes of incidence” — determine the overall level of a disease in a population.
Tobacco smoke offers a good example of how easy it is to mix them up. We know that someone exposed to tobacco smoke during childhood faces significantly higher odds of developing asthma. But we also know that tobacco smoke exposure can’t be responsible for the epidemic of asthma. The increase in asthma occurred precisely during times when the population prevalence of smoking was falling significantly. Air pollution tells the same story. Air quality improved substantially across many countries during the same period when rates of asthma were increasing. Two figures from Our World in Data show the historical data.
Put another way, these are important risk factors that influence how likely a given individual is to develop the disease. But they cannot be causative factors in the epidemic of asthma overall in the population because they demonstrate counter trends at the population level.
Primary and secondary risk factors in digital health
It’s natural for patients, physicians, and public health to assume the salient exposures they observe causing disease in individuals should be relevant and important for the population. But their perspective almost guarantees they will pay attention to secondary risk factors. Rose predicted this. He warned that patients and physicians were neither motivated nor capable of detecting the underlying risk factors of disease in a population. In fact, he noted that, in most cases, primary risk factors are not even evident until summed up across the population.
With its focus on the individual and his or her clinical care and treatment, digital health has similarly emphasized the experience of patients and physicians. And, in the last decade, we’ve learned a lot from technology about the (secondary) risk factors popular in clinical reports and case studies, but the evidence for their role in primary causation is weak.
In short, the widespread adoption of digital health has not translated into a better understanding of the causes of incidence, or led us to new preventive strategies. For the most part, we’re stuck with temporary, palliative public health measures. We have to continually focus on identifying and protecting the most susceptible individuals in the population because they will always be there.
For us to make progress at the population level — to make asthma and other chronic diseases less common in the first place — we need to figure out how to use technology to reveal or identify the underlying risk factors of the disease. One of the most important ways we might do that is by collecting vastly more and different types of data than we have ever had before.
Consider the traditional public health surveillance approach to asthma, designed largely to focus on analyzing information about the 30,000 or so sentinel events — hospitalizations and emergency room visits — that occur each week in the US. These are important outcomes but they represent a tiny fraction of the available information about asthma in the community. For example, we can assume there are between forty to fifty million uses of an albuterol inhaler every week across the country, all of them readily capturable and analyzable with distributed networks of sensors and connected medicines.
The bottom-up approaches of digital health bring the daily experiences of many more people—incl health, disease, environment, diet, social behavior—under statistical scrutiny. This means more opportunities to identify underlying primary risk factors, with other benefits such as greater timeliness, sample variability and geographic specificity, too.
But we need more than numbers. Rose noted the hardest cause to identify is the one universally present, because then it has no influence on the distribution of cases. He provided the example of the relationship between cardiovascular mortality and the overall hardness of the public water supply. Rose showed that in Scotland where everyone’s water is soft (left of the vertical, dotted line in the figure below), there appears to be no discernible relationship. The possibly adverse effect only becomes evident when you extend the study to areas with greater variation in exposure.
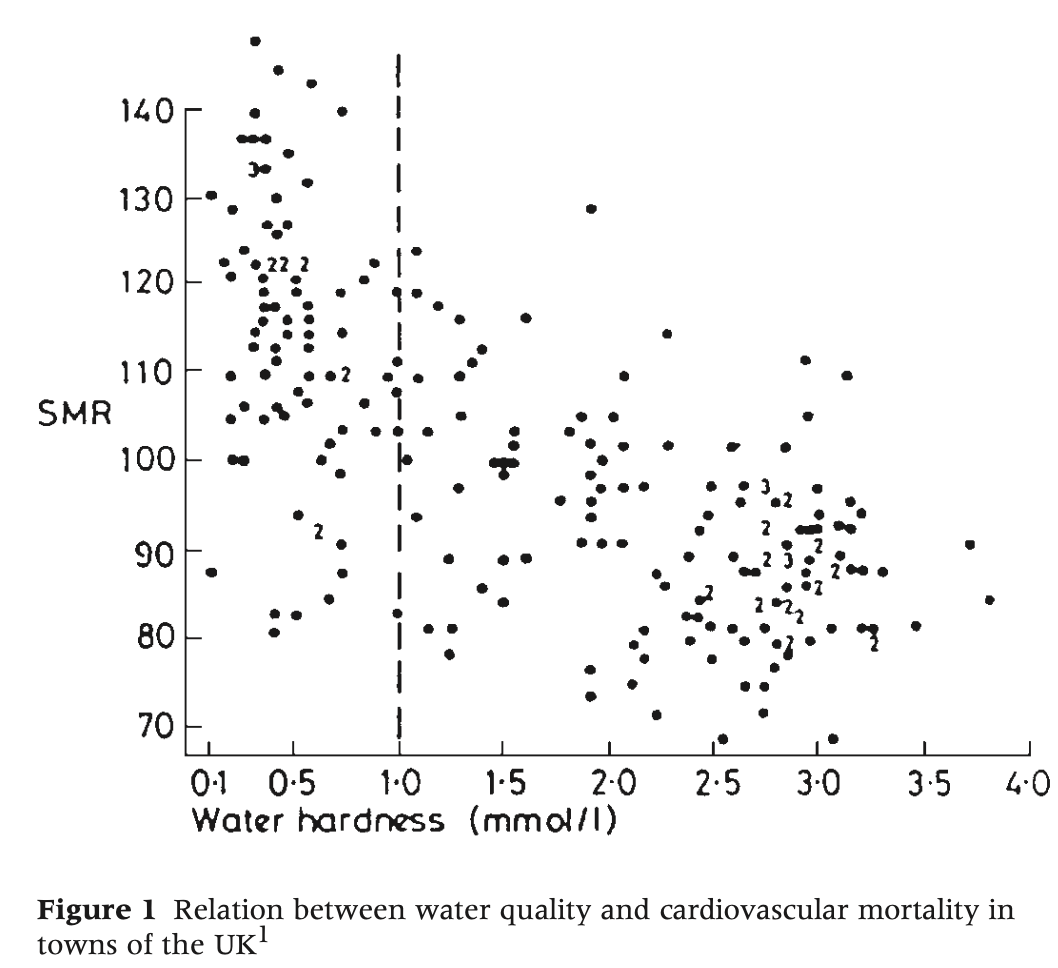
Rose pointed to tobacco use as another example. If everyone smoked twenty cigarettes, he explained, then every methodological tool we have — case control studies, clinical studies, cohort studies — would suggest that lung cancer was a genetic disease. And, in fact, they would be kind of right, for if the exposure to the necessary agent is uniform across the population then all that determines the distribution of cases is individual susceptibility.
Deliberately comparative research at scale
In the case of asthma and other chronic diseases, it’s probably too late to search for primary risk factors among the majority populations of high-income countries. In those settings, exposure to primary risk factors is likely already ubiquitous across the population, leaving us with just the effects of secondary risk factors. Fortunately this is one of the things that digital health is best suited to address.
With smartphones nearly universal, we can now involve many different types of people — populations that vary in prevalence, in exposures, and even those that abruptly change their lifestyle and environment. We can carry out international comparisons and cross-cultural experiments that have a greater probability of helping us understand primary risk factors underlying the cause of these diseases. Yet these efforts remain extraordinarily rare in digital health.
The healthtech industry can and should focus on improving day-to-day management, raising quality of life, and preventing individuals from developing disease when possible. But we should also aim for more fundamental victories. By rekindling and investing in an all-but-forgotten hunt for primary risk factors, we increase the chances that one day we can control the determinants of incidence, lower the mean levels of risk factors, and finally start to shift populations in a favorable direction.
Thank you for highlighting this important disconnect. I'd like to think digital health and all of the new kinds of data could help us "see" populations and individuals differently. And a big thank you for your partnership and risk taking that made our Louisville work such a good illustration. "We" improved individuals asthma control all the while seeing the effects of air pollution on the population. Amazing stuff.